How to Identify High-Impact AI Opportunities: A Guide for Business Leaders

Willem
CTO @ PromptArchitects
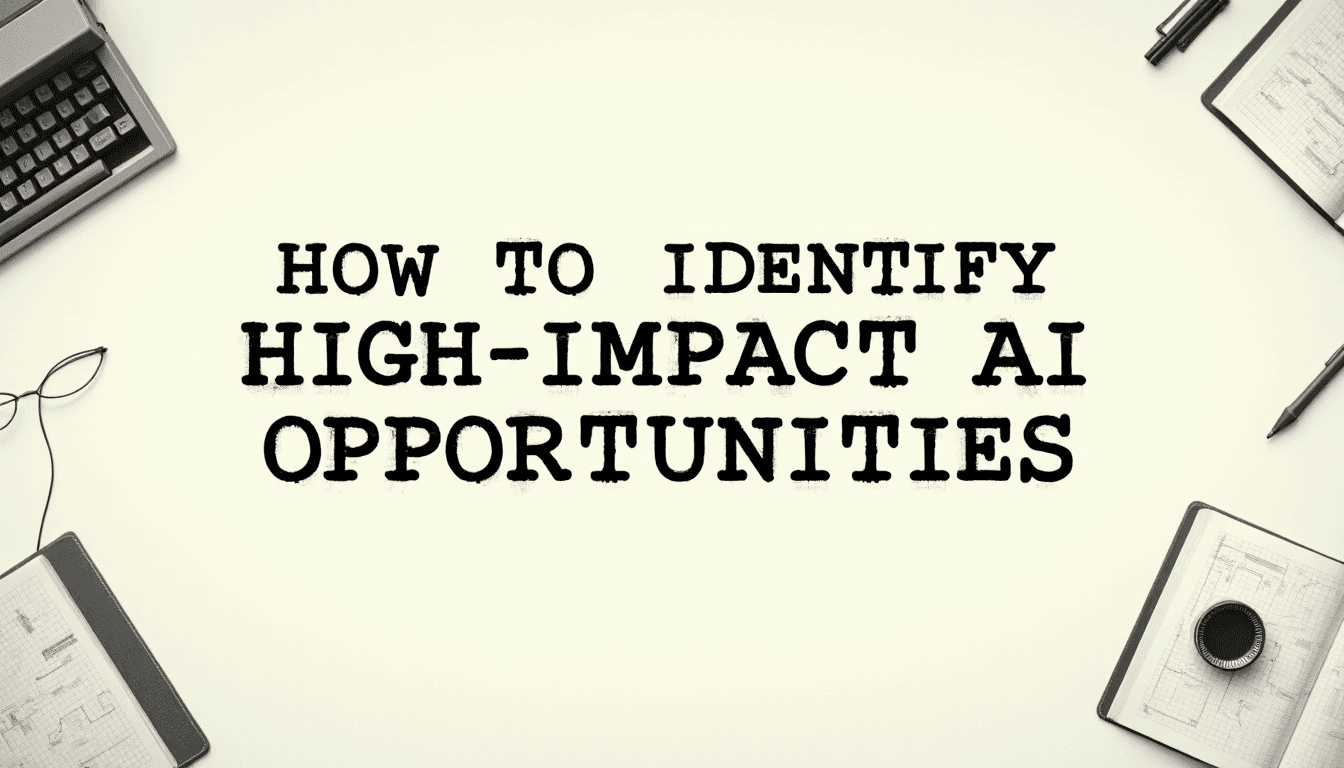
How to Identify High-Impact AI Opportunities: A Guide for Business Leaders
Are you ready to harness the power of AI for your business? As a leader, you know that staying ahead of the curve is crucial. AI isn't just a buzzword; it's a game-changer. Let's explore how you can spot and seize AI opportunities that will propel your business forward.
Understanding AI and Its Business Potential
AI is reshaping industries at breakneck speed. The global AI industry is expected to grow from $136 billion in 2023 to $803 billion in 2030, with a compound annual growth rate of 38.1%. This rapid growth could increase global productivity by 1.2% per year, potentially leading to a significant boost in living standards over the next few decades.
What is AI and How Does It Work?
At its core, AI is about machines mimicking human intelligence. It's the tech that powers voice assistants, recommends products, and even drives cars. AI systems learn from data, identify patterns, and make decisions with minimal human input.
Here's a simple breakdown:
- Data input: AI systems receive large amounts of data.
- Learning: They use algorithms to learn from this data.
- Pattern recognition: The AI identifies trends and patterns.
- Decision-making: Based on its learning, the AI makes decisions or predictions.
Current and Future AI Capabilities
Today, AI excels at:
- Processing natural language: Chatbots can handle customer inquiries 24/7.
- Recognizing images and speech: Security systems can identify potential threats.
- Making predictions based on data: Financial models can forecast market trends.
Tomorrow's AI might:
- Engage in complex problem-solving: Imagine AI tackling climate change solutions.
- Show emotional intelligence: AI therapists could provide mental health support.
- Create art and music indistinguishable from human-made works: AI could compose personalized songs for each listener.
Common AI Applications Across Industries
AI isn't just for tech giants. Here's how various sectors are using it:
- Retail: Personalizing shopping experiences
- Example: Amazon's recommendation engine suggests products based on browsing history.
- Healthcare: Assisting in diagnoses
- Example: AI analyzes medical images to detect early signs of cancer.
- Finance: Detecting fraud in real-time
- Example: Credit card companies use AI to flag suspicious transactions instantly.
- Manufacturing: Optimizing supply chains
- Example: Predictive maintenance reduces downtime in factories.
- Agriculture: Improving crop yields
- Example: AI-powered drones monitor crop health and optimize irrigation.
- Education: Personalizing learning experiences
- Example: Adaptive learning platforms adjust difficulty based on student performance.
"AI is not just a tool; it's a partner in innovation. It amplifies human creativity and problem-solving abilities." - Fei-Fei Li, AI researcher and professor at Stanford University
Assessing Your Organization's AI Readiness
Before diving in, let's check if your company is set for AI adoption.
Data Infrastructure Assessment
AI thrives on data. Ask yourself:
- Do we have enough quality data?
- Quantity: Aim for thousands or millions of data points.
- Quality: Is the data accurate, complete, and relevant?
- Is our data organized and accessible?
- Can different departments easily share data?
- Do we have a centralized data warehouse?
- Are we collecting the right data for our goals?
- Does our data align with our business objectives?
- Are there gaps in our data collection?
Pro tip: Start a data inventory. List all data sources, formats, and update frequencies.
Data Preparation and Quality Assurance
To ensure your AI project's success, focus on these key steps:
- Data cleaning: Remove inconsistencies, errors, and outliers.
- Data structuring: Organize data into a suitable format for AI analysis.
- Data preparation: Transform data for AI algorithms (e.g., normalization, feature engineering).
Technical Capabilities and Resources
Take stock of your tech muscle:
- Do we have staff with AI skills?
- Look for data scientists, machine learning engineers, and AI specialists.
- Is our IT infrastructure AI-friendly?
- Check if you have sufficient computing power and storage.
- Consider cloud computing options for scalability.
- Can we partner with AI experts if needed?
- Research potential AI consultants or technology partners.
Case study: A mid-size retailer partnered with a cloud AI provider to implement a recommendation system, saving time and resources on in-house development.
Cultural Readiness and Change Management
AI success hinges on people. Consider:
- Are our employees open to AI-driven changes?
- Conduct surveys to gauge attitudes towards AI.
- Share AI success stories from similar companies.
- How will we address fears about job displacement?
- Emphasize AI as a tool to enhance, not replace, human work.
- Plan for reskilling and upskilling programs.
- Do we have a plan to train staff on new AI tools?
- Budget for AI literacy programs across all levels.
- Identify AI champions within each department.
"The biggest barrier to AI adoption isn't technology; it's culture. Leaders must foster an environment of continuous learning and adaptation." - Andrew Ng, AI expert and founder of deeplearning.ai
Identifying High-Impact AI Opportunities
Now, let's uncover where AI can make the biggest splash in your business. We'll use a four-step framework to guide us through this process.
Step 1: Analyzing Business Processes and Pain Points
Start by looking inward:
- Which tasks eat up most of your team's time?
- Example: Manual data entry in finance departments.
- Where do errors frequently occur?
- Example: Order fulfillment mistakes in e-commerce.
- What processes have remained unchanged for years?
- Example: Traditional customer segmentation in marketing.
Real-world win: A logistics company found that route planning took hours daily. AI automation cut this to minutes, saving fuel and improving delivery times.
Action step: Create a process map of your business. Highlight areas with high manual input or frequent bottlenecks.
Step 2: Examining Customer Feedback and Needs
Your customers hold valuable clues:
- What complaints do you hear often?
- Example: Long wait times for customer support.
- Which services do customers wish you offered?
- Example: 24/7 account access in banking.
- How could you personalize experiences?
- Example: Tailored product recommendations in retail.
Real-world win: A bank implemented AI chatbots after noticing long wait times for customer service. Result? 24/7 support and happier customers.
Tip: Use sentiment analysis AI on customer reviews and social media mentions to uncover hidden insights.
Step 3: Conducting Market and Competitive Analysis
Don't reinvent the wheel. Research how others in your field use AI:
- Analyze competitors' AI initiatives
- What's working well for them?
- Where have they struggled?
- Look at industry leaders and innovators for inspiration
- Attend AI-focused industry conferences
- Read case studies from similar businesses
- Consider adopting best practices from other sectors
- AI solutions often have cross-industry applications
Resource: Join industry-specific AI groups on LinkedIn to stay updated on the latest trends and applications.
Step 4: Performing Feasibility Studies
Before committing to an AI project, assess its viability:
- Technical feasibility
- Do we have the necessary data and infrastructure?
- What technical requirements are needed for implementation?
- Financial feasibility
- What's the potential ROI?
- What are the upfront and ongoing costs?
- Operational feasibility
- How will this integrate with our current processes?
- What training will be required for staff?
Pro tip: Create a scoring system to rank AI opportunities based on feasibility and potential impact.
Brainstorming Sessions with Key Stakeholders
Gather your team's brainpower:
- Host an AI ideation workshop
- Invite representatives from different departments.
- Use design thinking methodologies to spark creativity.
- Encourage wild ideas – you can refine later
- Set a "no judgment" rule to encourage out-of-the-box thinking.
- Involve staff from different departments for diverse perspectives
- Include front-line employees who interact directly with customers.
Pro tip: Use a structured brainstorming method like "6-3-5 Brainwriting" to generate lots of ideas quickly.
Example exercise: "How might we use AI to solve our top 3 business challenges?"
Evaluating and Prioritizing AI Opportunities
You've got ideas. Now, let's sort the gold from the glitter.
Feasibility Analysis
For each AI opportunity, ask:
- Do we have the necessary data?
- Quantity, quality, and accessibility of relevant data.
- Can our current systems support this?
- Compatibility with existing IT infrastructure.
- What's the learning curve for our team?
- Estimate training time and resources needed.
Tool suggestion: Create a simple scoring system (1-5) for each criterion to compare opportunities objectively.
ROI and Value Proposition Assessment
Crunch the numbers:
- What costs will this AI solution cut?
- Example: Reduced labor costs, fewer errors.
- How much revenue could it generate?
- Consider direct sales increase and indirect benefits like improved customer satisfaction.
- What's the expected payback period?
- Aim for quick wins and long-term value.
Example calculation: Cost of AI implementation: $100,000 Annual savings: $50,000 Payback period: 2 years
Pro tip: Don't forget to factor in ongoing maintenance and update costs for AI systems.
Scalability Considerations
Think big:
- Can this AI solution grow with us?
- Consider future data volumes and business expansion.
- Will it work across different departments or locations?
- Look for solutions with flexible deployment options.
- How easily can we upgrade or modify it?
- Prefer modular AI systems that allow for easy updates.
Case study: A retail chain started with AI-powered inventory management in one store. The scalable solution allowed them to roll out to 500+ locations within a year.
Risk Assessment and Mitigation Strategies
Be prepared:
- What could go wrong?
- Consider technical failures, data privacy concerns, and ethical issues.
- How will we protect data privacy?
- Ensure compliance with regulations like GDPR or CCPA.
- What's our backup plan if the AI fails?
- Design fallback processes to maintain business continuity.
Create a simple risk matrix to visualize potential issues and their impact.
Risk mitigation strategies:
- Data privacy: Implement data anonymization or pseudonymization techniques.
- Technological limitations: Develop workarounds or alternative AI tools.
- Budget constraints: Create contingency plans for unexpected expenses.
Expert advice: "Always have a human-in-the-loop system for critical AI applications. It provides a safety net and builds trust." - Cynthia Breazeal, MIT Professor and AI ethics advocate
Creating an AI Implementation Roadmap
You've picked your AI projects. Now, let's plan for success.
Setting Clear Objectives and KPIs
Define what victory looks like:
- Specific: "Reduce customer churn by 15%"
- Measurable: "Increase production output by 1000 units per month"
- Time-bound: "Launch AI-powered recommendation engine in Q3"
Best practice: Align AI objectives with overall business goals. For example, if your company aims to improve customer satisfaction, set AI KPIs around reducing response times or increasing personalization.
Resource Allocation and Budgeting
Map out your needs:
- People: Data scientists, project managers, IT support
- Consider both in-house hiring and external consultants.
- Tech: Cloud computing resources, AI platforms, data storage
- Compare costs of building vs. buying AI solutions.
- Training: Courses for staff, workshops, onboarding programs
- Budget for ongoing AI literacy programs across the organization.
Tip: Start with a pilot project to gauge resource needs before scaling up.
Real-world example: A mid-size insurance company allocated 5% of its annual IT budget to AI initiatives, focusing on claims processing automation in year one.
Timeline and Milestone Planning
Break it down:
- Month 1-2: Data preparation and cleaning
- Audit existing data sources
- Implement data quality measures
- Month 3-4: AI model development and testing
- Select appropriate AI algorithms
- Conduct iterative testing and refinement
- Month 5: Staff training
- Develop training materials
- Conduct hands-on workshops
- Month 6: Pilot launch
- Deploy in a controlled environment
- Gather initial feedback
- Month 7-8: Evaluation and refinement
- Analyze pilot results
- Make necessary adjustments
- Month 9: Full rollout
- Implement across the organization
- Set up ongoing monitoring
Pro tip: Use agile project management methodologies for flexibility. AI projects often require iterative development and quick pivots.
Building Cross-Functional Teams
Assemble your AI dream team:
- Tech gurus who understand AI
- Data scientists and machine learning engineers
- Domain experts who know your business inside out
- Veteran employees with deep industry knowledge
- Change champions to drive adoption
- Influential team members from various departments
- Data analysts to feed the AI beast
- Experts in data preparation and visualization
Remember: Diversity in thought and background often leads to more innovative solutions.
Leadership insight: "The most successful AI teams I've seen blend technical expertise with business acumen. It's not just about building cool tech; it's about solving real business problems." - Cassie Kozyrkov, Chief Decision Scientist at Google
Implementing and Monitoring AI Solutions
It's showtime. Let's get your AI up and running.
Pilot Projects and Proof of Concepts
Start small, learn fast:
- Choose a contained area for your first AI project
- Example: Implement a chatbot for a specific product line.
- Set a clear timeframe, like 6-8 weeks
- Define what success looks like at the end of the pilot.
- Gather feedback relentlessly
- Set up regular check-ins with users and stakeholders.
Success story: A retailer tested an AI inventory system in one store before rolling out company-wide, ironing out kinks and building confidence.
Tip: Create a feedback loop with end-users. Their insights are gold for refining your AI solution.
Data Preparation and Model Selection
Garbage in, garbage out:
- Clean your data meticulously
- Remove duplicates, handle missing values, and standardize formats.
- Choose AI models that fit your specific needs
- Consider factors like interpretability, speed, and accuracy.
- Consider pre-trained models to save time and resources
- Many cloud providers offer ready-to-use AI models for common tasks.
Data quality checklist:
- Is it accurate?
- Is it complete?
- Is it consistent across sources?
- Is it timely and up-to-date?
Integration with Existing Systems
Smooth sailing is key:
- Ensure AI tools play nice with your current tech stack
- Test integrations thoroughly before full deployment.
- Plan for data flow between systems
- Set up secure, efficient data pipelines.
- Train staff on new workflows
- Create user-friendly guides and offer hands-on training sessions.
Case study: A healthcare provider successfully integrated an AI diagnostic tool with their existing electronic health record system, improving diagnosis speed without disrupting doctors' workflows.
Continuous Monitoring and Improvement
Keep your finger on the pulse:
- Set up real-time monitoring dashboards
- Track key performance metrics and system health.
- Schedule regular performance reviews
- Monthly or quarterly deep dives into AI performance.
- Be ready to tweak and optimize on the fly
- AI models can "drift" over time. Plan for regular retraining.
Best practice: Implement A/B testing for AI improvements. Compare the performance of updated models against the current version before full deployment.
Case Studies: Successful AI Implementations
Let's look at some AI wins across different sectors.
Finance: Fraud Detection and Risk Management
A major credit card company implemented AI to spot fraudulent transactions. Result? They caught 99% of fraud attempts, saving millions and boosting customer trust.
Key takeaways:
- Real-time processing of vast transaction data
- Continuous learning from new fraud patterns
- Reduced false positives, improving customer experience
Healthcare: Diagnosis and Treatment Planning
An AI system analyzed thousands of retinal images, detecting early signs of diabetic retinopathy with 90% accuracy. This allowed for earlier treatment and better outcomes.
Impact:
- Faster screenings, enabling more patients to be tested
- Reduced workload for ophthalmologists
- Improved access to care in underserved areas
Retail: Personalization and Inventory Management
A fashion retailer used AI to predict trends and manage stock. They reduced overstock by 20% and increased sales by 15% through personalized recommendations.
How they did it:
- Analyzed social media trends and historical sales data
- Implemented dynamic pricing based on demand
- Created personalized "style profiles" for customers
Manufacturing: Predictive Maintenance and Quality Control
A car manufacturer implemented AI-powered predictive maintenance. They cut downtime by 30% and slashed maintenance costs by $5 million annually.
Key components:
- Sensors on manufacturing equipment to collect real-time data
- AI models to predict equipment failures before they occur
- Integration with inventory systems for just-in-time parts ordering
Agriculture: Precision Farming
A large farm implemented AI-driven precision agriculture techniques. They increased crop yields by 15% while reducing water usage by 20%.
Technologies used:
- Drone imagery for crop health monitoring
- AI-powered weather prediction for optimal planting and harvesting times
- Automated irrigation systems based on soil moisture sensors
"These case studies show that AI isn't just for tech companies. Any industry can find innovative ways to apply AI and reap significant benefits." - Erik Brynjolfsson, Director of the Stanford Digital Economy Lab
Addressing Common Questions and Misconceptions
Let's clear the air on some AI confusion.
What is AI Identification?
AI identification is about recognizing objects, faces, or patterns. It's how your phone unlocks when it sees your face or how a self-driving car knows a stop sign from a yield sign.
Real-world applications:
- Security systems using facial recognition
- Medical imaging for detecting abnormalities
- Quality control in manufacturing to spot defects
How to Identify AI-Generated Content
Spotting AI-created content is tricky, but look for:
- Unusual phrasing or repetition
- Lack of personal anecdotes or specific examples
- Inconsistencies in tone or style
Remember: AI content detection tools exist, but they're not foolproof.
Tip for leaders: Focus on the value of the content, whether human or AI-generated, rather than its origin.
Ethical Considerations in AI Implementation
As you embrace AI, keep ethics front and center:
- Ensure AI decisions are explainable and fair
- Implement "explainable AI" techniques to understand how decisions are made.
- Protect user privacy fiercely
- Follow data protection regulations and implement strong security measures.
- Consider the broader impact on society and jobs
- Plan for reskilling programs and responsible AI deployment.
"AI ethics isn't just about avoiding harm. It's about actively designing systems that promote fairness, transparency, and human values." - Timnit Gebru, AI ethics researcher
Future Trends in AI Technology
Keep an eye on these emerging AI frontiers:
- Quantum AI: Solving complex problems at mind-boggling speeds
- Potential to revolutionize drug discovery and financial modeling
- Edge AI: Running AI on devices, not in the cloud
- Enables faster, more private AI applications on smartphones and IoT devices
- AI-human collaboration: Tools that enhance, not replace, human skills
- Think AI assistants that augment human creativity and decision-making
"The future of AI is not about machines taking over, but about humans and AI working together to solve problems we couldn't tackle alone." - Kai-Fu Lee, AI expert and venture capitalist
Conclusion: Taking the Next Steps
You're now armed with knowledge to spot and seize AI opportunities. Here's your action plan:
- Assess your AI readiness
- Conduct a thorough data and skills audit
- Identify your most pressing business challenges
- Engage with frontline staff and customers
- Explore how AI could address these issues
- Research industry-specific AI applications
- Start small with a pilot project
- Choose a high-impact, low-risk area for your first AI implementation
- Learn, adjust, and scale
- Use insights from your pilot to refine your AI strategy
Remember, the AI journey is a marathon, not a sprint. Stay curious, be bold, and keep learning. The future is AI-powered, and you're now ready to lead the charge.
Final thought: "The best way to predict the future is to create it. With AI, you have the tools to shape your industry's tomorrow. The question is, what future will you build?" - Peter Diamandis, founder of XPRIZE Foundation
Ready to turn your AI vision into reality? Let's talk about how we can help you build a game-changing AI solution for your business. Contact us for a free AI readiness assessment and start your journey to becoming an AI-powered organization today.
Key AI adoption statistics:
- 72% of businesses expect to incorporate AI into their processes within the next 3 years.
- AI-powered fraud detection solutions could save businesses $31 billion annually by 2024.
- AI can help businesses reduce supply chain costs by up to 15%.
- 76% of consumers are more likely to buy from companies offering personalized messaging and services.
- AI could boost global GDP by $13 to $15 trillion annually by 2030, equivalent to an additional 1.1% to 1.4% GDP growth per year.
By embracing AI opportunities strategically, you're not just keeping up with the competition – you're positioning your business to thrive in an AI-driven future. The time to act is now. Are you ready to lead the AI revolution in your industry?